Either we are not that special and unique, or our AI is far more capable of acquiring human language quirks.
Psycholinguistics is about understanding language from the behavioral point of view. It’s about understanding the patterns across languages. I made a small introduction before, but you can directly start here as a deeper look at psycholinguistics (as defined by humans for humans) and applied to AI.
I read a pre-print of a paper recently that made me delete a huge claim I was going to make in my upcoming book – I was going to claim that AI is incapable of developing human-like language quirks on its own. When I told my friends about their discoveries, they were surprised that the boundary between Humans and AI is becoming quite blurry.
The paper, Do large language models resemble humans in language use?[1], by multiple researchers from the Department of Linguistics and Modern Languages, The Chinese University of Hong Kong, Brain and Mind Institute, The Chinese University of Hong Kong, and the Department of Psychology, University of Edinburgh.
They tested 2 AI models: ChatGPT 4o (openAi) and Vicuna (an open-source model), on 12 psycholinguistic properties of human languages. They compared the 2 models with human performance.
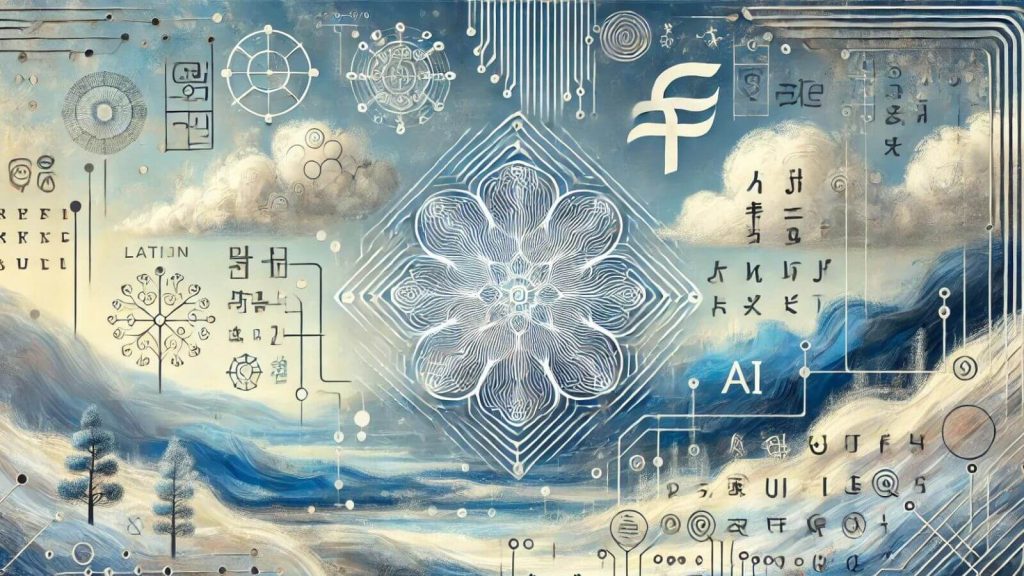
I won’t get into the technical details of the model’s performance, but I’ll highlight how LLMs (ChatGPT and Vicuna) fare against humans.
- Sound-Shape Association
- Sound-Gender Association
- Word Length and Predictivity
- Word Meaning Priming
- Structural Priming
- Syntactic Ambiguity Resolution
- Interpreting Implausible Sentences
- Semantic Illusion
- Implicit Causality
- Drawing Inferences
- Interpreting Ambiguous Words by Context
- Choosing Context-dependent Words
- Sources
Sound-Shape Association
People tend to associate certain sounds with certain shapes. For example, novel words like “Kiki” are associated with spiky shapes, while “Bouba” is associated with round shapes. Humans do this by finding some similarity between the sound patterns & the visual patterns. The similarity is first sensory. But we also do this conceptually more often – we call them metaphors.
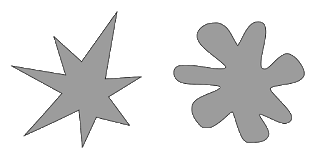
AI performance: Both ChatGPT and Vicuna assigned round-sounding novel words to round shapes more often than spiky shapes. ChatGPT showed a stronger association than Vicuna.
One reason this is happening is that word tokenizations have discovered this pattern because tokenization is not limited to single words, it is done for word components. Pair this with the theory of how humans do this in its training data, and the sound-shape association will emerge from the billions of parameters. The only difference – and may be the biggest one is – humans (and babies) and even chimps can do this by default without training, but AI learns this through extensive training.
LLMs are text and vision-centric right now. The moment more sensory modalities are available, it is likely that AI becomes excellent at all similar associations – sound/shape, taste/sound, color/name, etc. This is the study of cross-modal correspondence, which is also my first area of research.
Verdict: AI is capable of sound-shape associations. This is very, very impressive because we had always assumed this to be a unique human property.
Sound-Gender Association
People can guess the gender associated with unfamiliar names based on their sounds. For example, names ending in vowels are often perceived as feminine. This tendency is seen across many cultures with gender associations to words. Linguistically, softer and rounder endings tend to be feminine, and those with harsher and consonant endings tend to be masculine. A lesser-known fact is that the idea of gender comes from the concept of “genus” meaning “category” or “type”. Genders existed before we applied them to human identity and then used those identity references as the popular understanding of gender.
AI Performance: Both models were more likely to use feminine pronouns for vowel-ending names than consonant-ending names. ChatGPT showed a stronger association than Vicuna.
Verdict: AI is expected to discover this pattern and apply it using tokenization if it is given a large data set containing such references.
Word Length and Predictivity
We choose shorter words more often when their meanings are predictable from the context. Example: Choosing “math” instead of “mathematics” in a predictive context like “Susan was very bad at algebra, so she hated math/mathematics.” Humans tend to use the short form because it is processed more easily.
AI Performance: Neither model exhibited a significant tendency to choose shorter words in predictive contexts.
Verdict: Perhaps AI doesn’t have the ease-of-processing concept for its own self-regulation because it is currently spending computational resources based on humans monetizing it. If the AI was forced to operate within its own means, the AI should choose and minimize effort, just like humans do.
Word Meaning Priming
People tend to access the more recently encountered meaning of an ambiguous word. This plays into the recency bias or availability heuristic – which states that whatever is more recently learned or noticed is more available in the brain for future use.
For example, if 2 people have a conversation about the word “post” and one has recently looked for a job (aka job post) and the other made a social update (posted online), both will have different mental responses to the word before they realize the context of a 3rd person talking about a goal post.
AI Performance: Both models accurately interpreted the ambiguity using a prior context.
Verdict: Humans understand context through situational variables, prior conversations, expected events, etc. AI understands them through word associations primarily, but prior information allows correct interpretation. The behavior mimics humans, but I’ll still say that this is a very easy task about context. Not that impressive.
Structural Priming
People tend to repeat a syntactic structure that they have recently encountered. Once we start speaking in the active voice or passive voice, following sentences tend to maintain that sentence structure.
Using the same syntactic structure in “The patient showed his hand to the nurse” after a similar structure like “The racing driver gave the torn overall to his mechanic.”
AI Performance: Both models showed structural priming, with a stronger effect when the prime and target had the same verb.
Verdict: AI must’ve acquired sentence structures as a method to generate text. Once it is acquired, it is probably applied to the following token predictions. That means the LLM has already set its “voice” unless instructed otherwise.
Syntactic Ambiguity Resolution
People use context to resolve syntactic ambiguities. This form of context comes from predicting how things happen in general. That means, overall speaking, listening, and reading experience teaches us what to expect and when to expect a certain way a story ends.
For example, interpreting “The hunter shot the dangerous poacher with the rifle” as the poacher having the rifle vs. the hunter having the rifle. Humans who think poachers are being illegal would assume the hunter did the right thing. Humans who think people with power abuse it might think the hunter over-exercised their power and used their licensed rifle illegally.
AI Performance: Neither model used context to resolve syntactic ambiguities as humans do.
Verdict: YAY humans. This sort of context requires a wide understanding of human experience, situations, and perspective. It involves knowing how things happen, how they shouldn’t, and when expectations get violated. Current methods of understanding context in AI (the contextual window of a token size, beam search) are insufficient. But the newer developments called EM-LLM[2] (episodic memory LLM) promise to give infinite context to LLM by treating context as separate episodic experiences similar to how humans store memories as “episodic memories”. They are essentially holistic experiences that are stored as large associative networks of whole memories and individual memory components.
Interpreting Implausible Sentences
People nonliterally interpret implausible sentences to understand intended meanings.
For example, humans can correctly interpret a wrong sentence like “The mother gave the candle the daughter” as the daughter received a candle, not that the candle received the daughter.
AI Performance: ChatGPT, but not Vicuna, made more nonliteral interpretations for implausible sentences, showing sensitivity to syntactic structure.
Verdict: YAY humans. AI doesn’t yet have the concept of reality-grounding – things that cannot be possible or are physically non-sensical. Humans are governed by physical interactions and the limits of physics and the environment. Easy for humans, but difficult for AI. And possibly, difficult for people who aren’t fluent in a language – lack of fluency might make the listener doubt their understanding first before being confident that the sentence is implausible.
Semantic Illusion
People often fail to notice conspicuous errors in sentences when the error is semantically close to the intended word. For example, humans will quickly understand “I was drinking beer” even if the word beer is replaced by “bear.” The same happens with semantically similar confusion like mixing up dog and cat. E.g., my dog was purring when he heard footsteps.
AI Performance: ChatGPT showed a humanlike tendency to gloss over errors caused by semantically similar words, while Vicuna did not.
Verdict: This shows that AI is somewhat capable of understanding concepts in a context but might fail at understanding typical vs. non-typical situations. Not bad, and we’ll likely see very strong improvements as we develop newer techniques to teach AI concepts apart from just word associations.
Implicit Causality
We ascribe causality in sentences based on the verb’s thematic roles. E.g., the ball broke the window pane. The cause is understood as the ball caused the breakage. But likely also another cause – someone threw the ball or hit the ball with a bat in the wrong direction. We also see clear causes within a sentence’s structure using verbs – Attributing causality to the object in “Gary feared Anna because she was violent.” Anna is the object, Gary is the subject. Feared is the verb. And violent paired with Anna gives context to the verb “feared”.
AI Performance: Both models showed sensitivity to the semantic biases of verbs, attributing causality similarly to humans.
Verdict: Given enough examples and text comprehension lessons, this is expected. LLMs are getting very good at this and are being as to generate text-comprehension questions for education.
Drawing Inferences
People make inferences to connect information. 2 sentences can give a logical flow to a larger story or additional sentences can fill in the missing gaps. Example: inferring that Sharon cut her foot after reading “Sharon stepped on a piece of glass. She cried out for help.”
AI Performance: ChatGPT, but not Vicuna, was more likely to draw such conclusions.
A likely explanation is that this is an emergent property of training data, not exactly a critical thinking ability. An unknown here is if there are specific “causality” markers within those billions of parameters. Specialized AI for solving math, physics, and chemistry problems may be trained to understand causality and logical flow. But, whether LLMs have that capacity as an ability, or it is an emergent property of how language is used in its training data – remains unknown.
Verdict: Seems simple for humans, but the set of assumptions people make are contextually rich and depend a lot on past experience and what’s possible in a physical world. AI will have a hard time. But if AI can overcome this with just examples and extract its own form of logic in it, I’d say it is great progress.
Interpreting Ambiguous Words by Context
We interpret words to mean a specific thing based on the cultural/social background of others. For example, we might interpret “bonnet” as “car part” when the interlocutor is a British English speaker.
AI Performance: Both models accessed meanings more aligned with the interlocutor’s dialectal background.
Verdict: This is brilliant and extracting such contextual ideas from words is great. But, humans rely on more than words – visuals, speech intonation, clothes, location, etc. But again, since LLMs are limited to words, performing as well as humans with just words is pretty much ideal. It’s just like a simple version of what we do, simply because the LLMs taken is simpler forms of data.
Choosing Context-dependent Words
People retrieve words based on the interlocutor’s dialectal background during language production. When we respond to others, we choose words appropriate to others’ likely interpretation of that word.
For example, we’ll probably say “apartment” instead of “flat” when the listener is an American English speaker and “flat” if it is an Indian English speaker.
AI Performance: Both models retrieved words more aligned with the interlocutor’s dialectal background.
Verdict: The same as above. The only difference is interpretation (meaning access) vs. retrieval (decision-making). So……I’ll repeat myself. Humans rely on more than words – visuals, speech intonation, clothes, location, etc. But again, since LLMs are limited to words, performing as well as humans with just words is pretty much ideal. It’s just like a simple version of what we do, simply because the LLMs take in simpler forms of data.
On the surface, it appears that LLMs should have these quirks, but the excitement is in how AI acquires these – Some quirks are learned, and many are re-discovered within its processes.
Sources
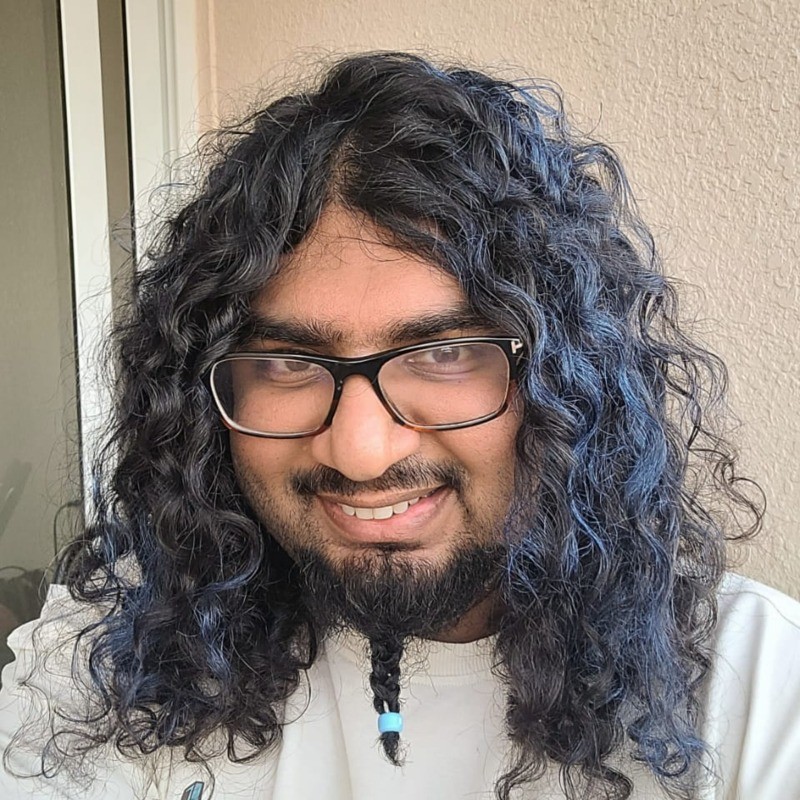
Hey! Thank you for reading; hope you enjoyed the article. I run Cognition Today to capture some of the most fascinating mechanisms that guide our lives. My content here is referenced and featured in NY Times, Forbes, CNET, and Entrepreneur, and many other books & research papers.
I’m am a psychology SME consultant in EdTech with a focus on AI cognition and Behavioral Engineering. I’m affiliated to myelin, an EdTech company in India as well.
I’ve studied at NIMHANS Bangalore (positive psychology), Savitribai Phule Pune University (clinical psychology), Fergusson College (BA psych), and affiliated with IIM Ahmedabad (marketing psychology). I’m currently studying Korean at Seoul National University.
I’m based in Pune, India but living in Seoul, S. Korea. Love Sci-fi, horror media; Love rock, metal, synthwave, and K-pop music; can’t whistle; can play 2 guitars at a time.